The Ineffective Artificial Intelligence: Understanding Predictive Analytics vs. Artificial Intelligence
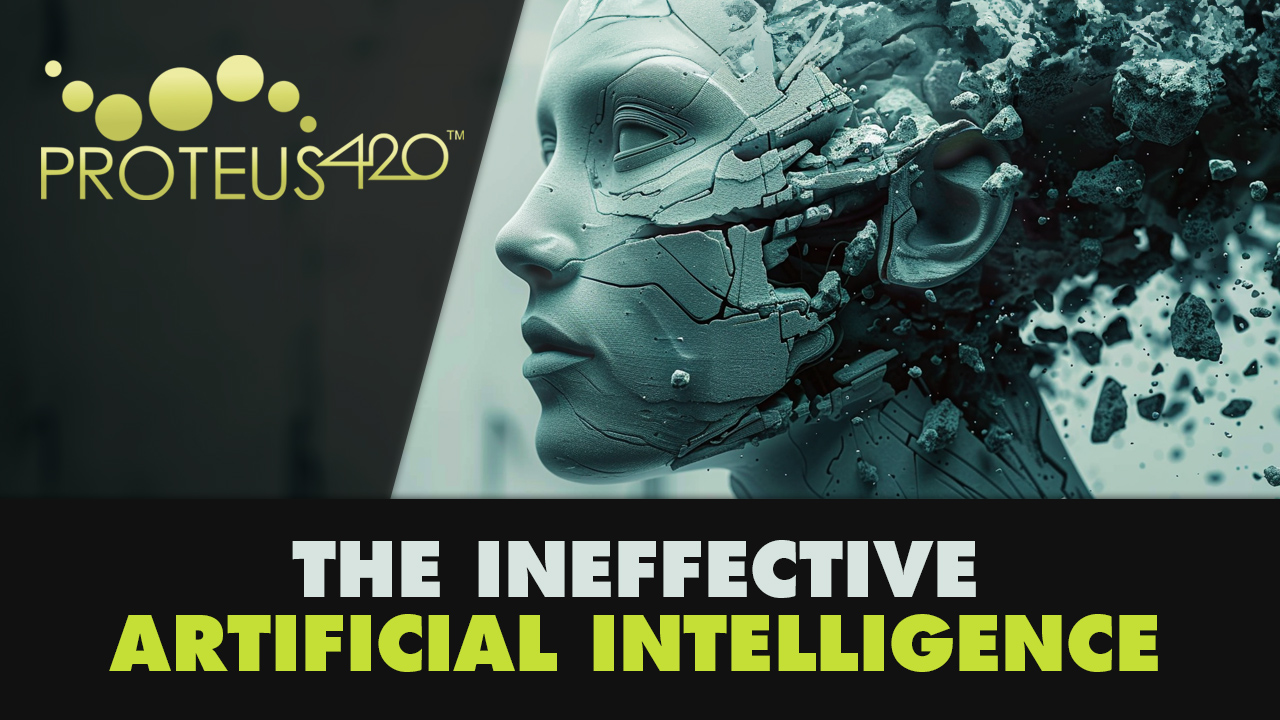
In the rapidly evolving tech landscape, Artificial Intelligence (AI) and Predictive Analytics are often spotlighted as transformative forces driving innovation and efficiency. Despite their prominence, there is a common misconception that these technologies are similar, or even interchangeable. However, this is far from the truth. Predictive analytics is a discipline focused on extracting information from existing data sets in order to forecast future probabilities. AI, in contrast, encompasses a broader spectrum of capabilities, including but not limited to predictive functionalities. This article aims to clarify the distinct roles and functionalities of predictive analytics and artificial intelligence, demonstrating why equating the two can lead to misguided applications and ineffective AI. By understanding their unique attributes and applications, organizations and individuals can better harness the specific strengths of each technology to drive success and innovation.
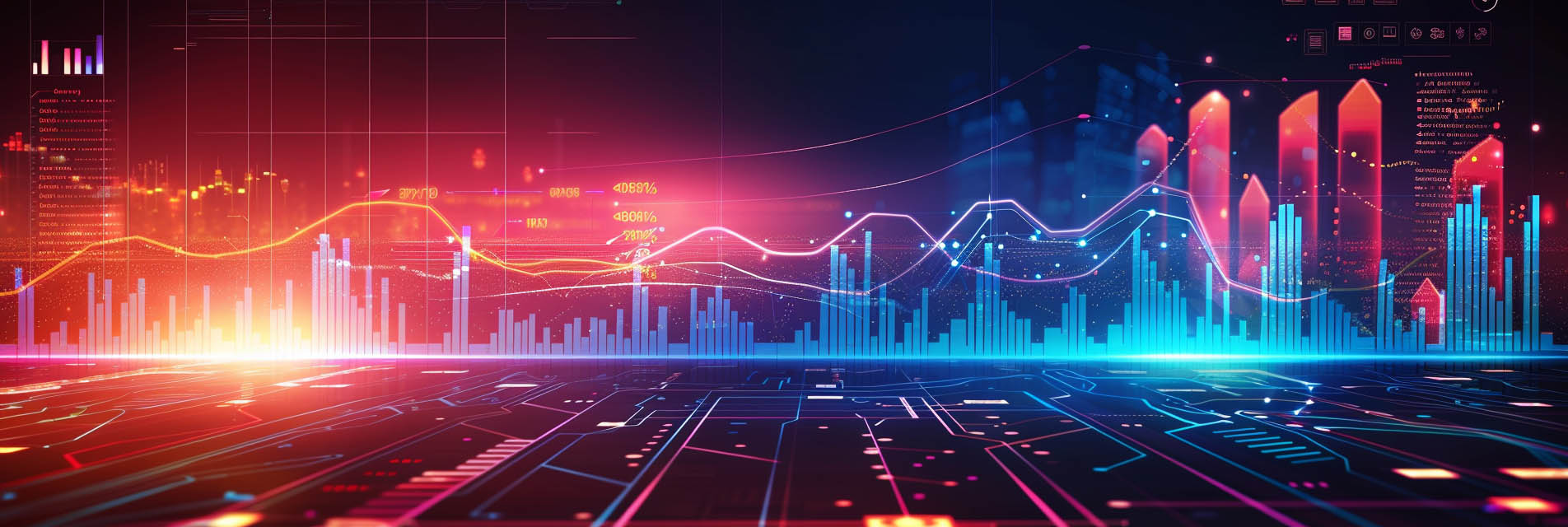
Understanding Predictive Analytics
Predictive analytics is a statistical technique used across various industries to make informed guesses about future events based on historical data. By integrating methods such as data mining, statistical modeling, and machine learning, predictive analytics helps organizations anticipate outcomes and trends.
Core Components of Predictive Analytics:
- Data Collection: Gathering raw data from multiple sources which is cleaned and prepared for analysis.
- Statistical Analysis: Applying statistical techniques to understand past behaviors and trends.
- Predictive Modeling: Creating models that use past data to predict future outcomes. Common models include regression analysis, time series analysis, and clustering.
Applications in Real Life:
- In finance, predictive analytics is used to assess credit risk or to forecast stock market trends.
- In healthcare, it can predict patient outcomes based on treatment plans or anticipate future admission rates.
- Retail businesses apply predictive analytics to determine customer purchasing behavior or optimize inventory levels based on predicted demand.
- By leveraging these techniques, predictive analytics provides actionable insights that help businesses optimize operations and reduce risks. However, it is crucial to understand that predictive analytics is inherently limited to making forecasts based on existing patterns; it does not possess the capability to understand or interpret data beyond its programming.
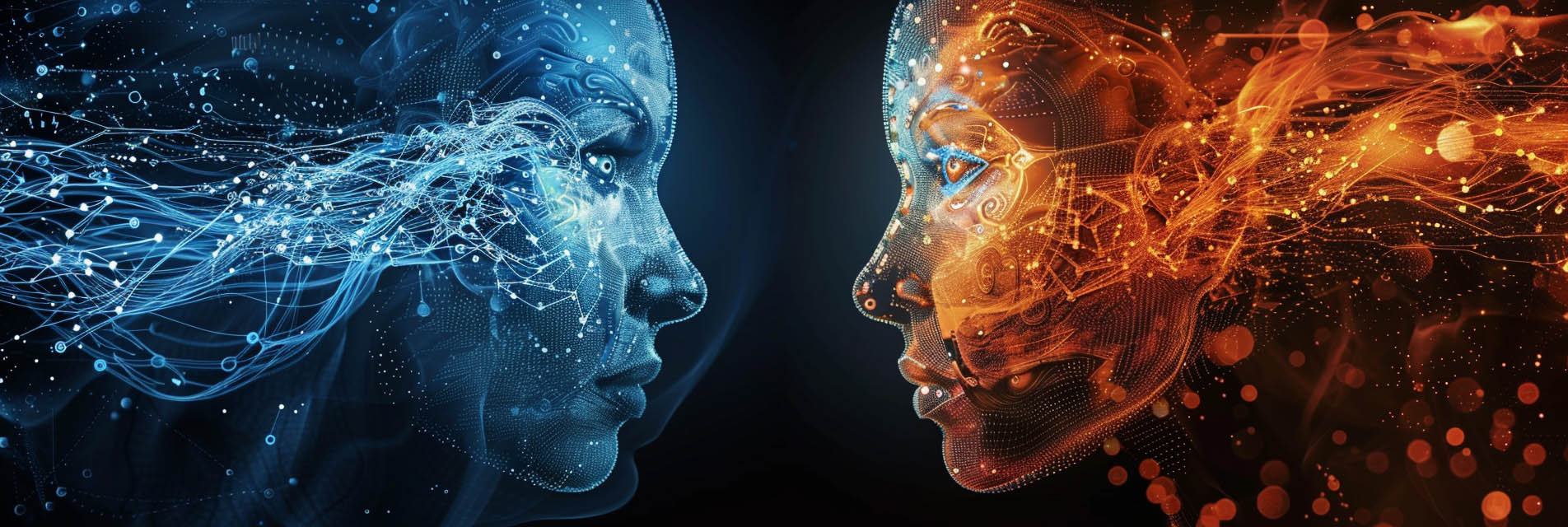
Exploring Artificial Intelligence Artificial Intelligence (AI) represents a vast field that stretches well beyond the realms of predictive analytics. At its core, AI involves creating systems that can perform tasks typically requiring human intelligence. These tasks range from recognizing speech, and interpreting complex data, to making decisions in uncertain environments.
Key Elements of AI: Machine Learning (ML): This is where algorithms learn from and make predictions on data, improving over time without being explicitly programmed. Deep Learning: A subset of ML, deep learning utilizes neural networks with many layers to analyze various factors of large data sets. Natural Language Processing (NLP): AI's ability to understand and interact using human language, applied in tools like chatbots and translation services. Broader Applications of AI:
- Autonomous Vehicles: Using AI to interpret sensory information to navigate safely through various environments.
- Healthcare Diagnosis: AI systems analyze complex medical data to assist in diagnosis and treatment plans, often recognizing patterns that are not obvious to human observers.
- Robotics: Robots equipped with AI can perform tasks ranging from precision surgery to industrial assembly with high efficiency and adaptability.
Unlike predictive analytics, AI is not limited to making predictions based on historical data. AI can simulate understanding and adapt to new situations, learn from new data in real-time, and make decisions autonomously. This broader capability allows AI to operate in dynamically changing environments, providing solutions that are not merely forecasts but informed decisions made through understanding and reasoning.
Comparative Analysis: Apples and Oranges
Understanding the differences between predictive analytics and artificial intelligence is crucial for utilizing each technology effectively. While they intersect, their applications, capabilities, and underlying technologies have distinct characteristics that set them apart.
Fundamental Differences:
Scope of Application: Predictive analytics is specifically designed for forecasting future events based on past data. AI, however, spans a much broader range of cognitive functions, including learning, reasoning, problem-solving, and understanding human language.
Capability for Learning and Adaptation: Predictive analytics operates within the confines of its predefined algorithms and models. AI, particularly through machine learning and deep learning, adapts and learns from new data without human intervention, continuously improving its accuracy and effectiveness.
Technological Complexity: The models used in predictive analytics are generally less complex than those driving AI systems. AI involves sophisticated algorithms that can process and analyze data in a more nuanced and comprehensive manner.
Illustrative Examples:
Predictive Analytics: A retail chain uses customer purchase history to forecast future buying trends and manage inventory. This approach relies strictly on patterns and trends identified from past behavior.
Artificial Intelligence: An AI-powered virtual assistant uses natural language processing to understand spoken language, learn from interactions, and provide responses that adapt to the user’s needs and preferences over time.
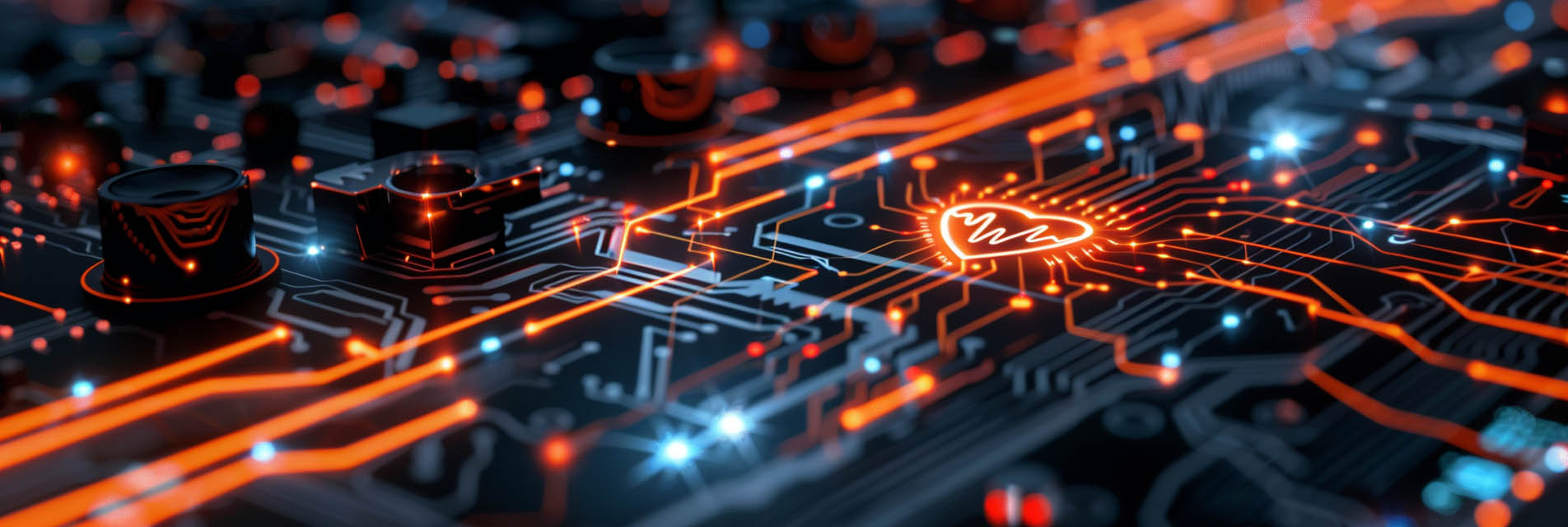
Expectations vs. Implementations Pitfalls of Conflation:
Conflating predictive analytics with AI can lead to underutilization of advanced AI capabilities or misapplication where simpler predictive models might suffice. Misunderstanding these distinctions can lead to unrealistic expectations and ineffective implementations, where the tools are not aligned with their intended purposes.
Case Studies Highlighting MisapplicationsExploring real-world misapplications of predictive analytics and AI can provide valuable lessons on the importance of distinguishing between these two technologies. Here are a couple of case studies that illustrate the pitfalls of conflating predictive analytics with AI.
Case Study 1: Healthcare Misdiagnosis Scenario: A medical institution implemented a system they believed utilized AI to diagnose diseases. The system was actually based on simple predictive models that used historical patient data to predict health outcomes.
Problem: The system failed to account for rare, atypical symptoms not well-represented in the historical data, leading to several misdiagnoses. Outcome: The misapplication of basic predictive analytics instead of more sophisticated AI led to inadequate patient care and trust issues among the medical staff. Case Study 2: Retail Inventory Mismanagement Scenario: A large retail chain used what they thought was an AI-driven tool to manage inventory and forecast demand. Problem: The tool was strictly a predictive analytics model that did not adapt to sudden market changes or incorporate real-time data from social media and current events. Outcome: The retailer experienced overstocks of some products and shortages of others, resulting in significant financial losses and damaged customer relations.
Lessons Learned:These examples highlight the necessity for clarity in the capabilities and limitations of the technology being implemented. Predictive analytics is powerful for projecting trends based on historical data, but it lacks the adaptive, self-learning capabilities of true AI, which can analyze complex patterns and adjust to new information in real-time.
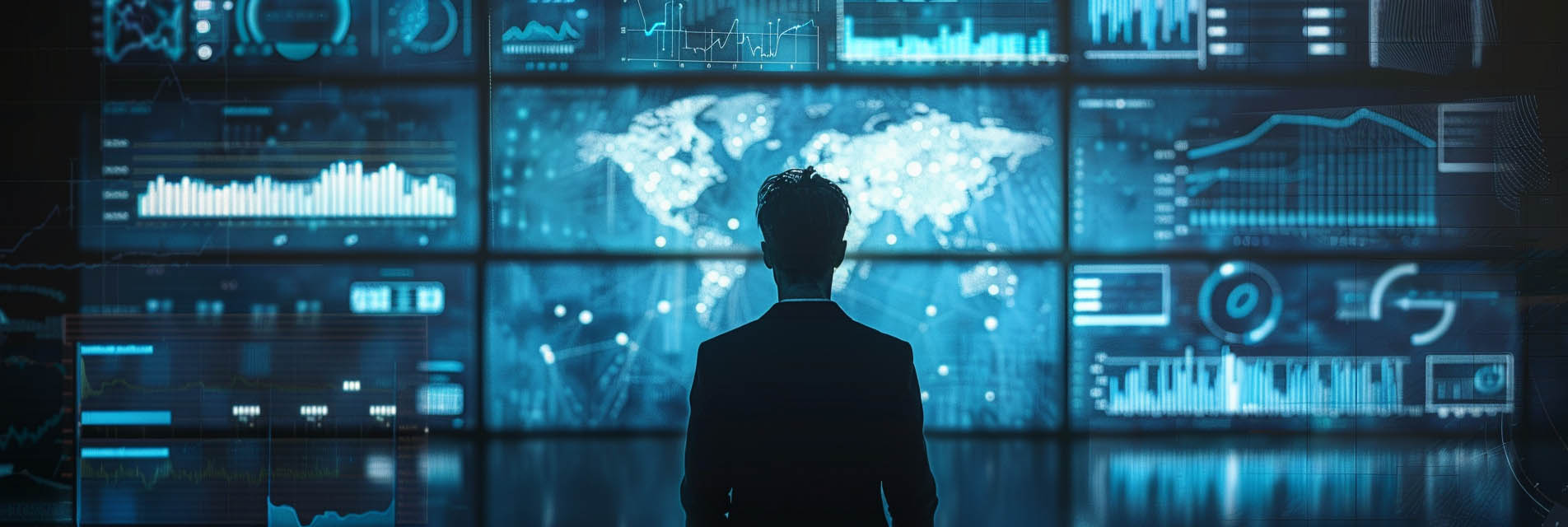
The distinction between predictive analytics and artificial intelligence is not just academic; it has practical implications for the effectiveness of technology applications in business, healthcare, and many other sectors. Predictive analytics is a powerful tool for forecasting based on historical data, but it does not encompass the broader, more dynamic capabilities of AI, which include learning from new data, adapting to changing environments, and performing tasks that typically require human intelligence.
To leverage the full potential of these technologies, it is crucial to understand their unique strengths and limitations. Organizations that recognize the differences can make informed decisions about which technology to deploy in various scenarios, leading to more effective outcomes and avoiding the pitfalls of misapplication.
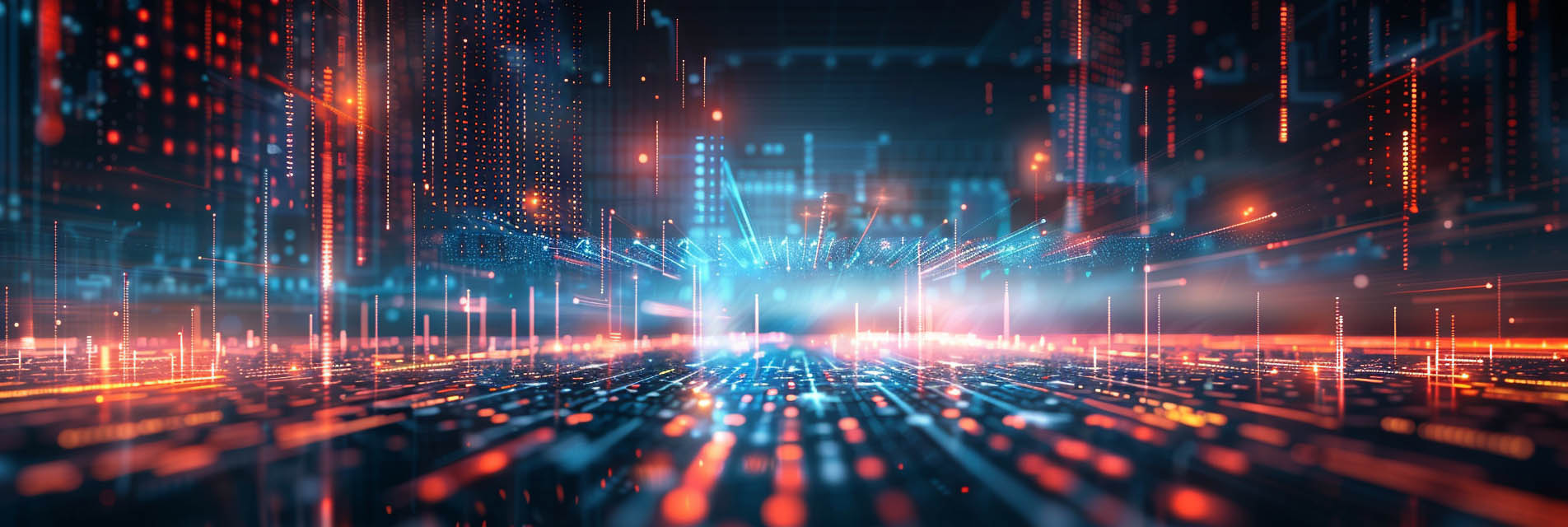
As we continue to advance into a data-driven future, the clarity in these distinctions will be paramount in fostering innovations that are not only technologically advanced but also aligned with the practical needs of industries looking to adopt these tools. Further exploration and education in the fields of AI and predictive analytics will help clarify their roles and ensure they are used effectively to drive progress.